Are your production processes inefficient, leading to high costs and low productivity? Is it difficult to manage your inventory and predict supply chain disruptions? Do you struggle with maintaining consistent product quality?
These are common pain points for many manufacturers. Fortunately, data-driven decision-making can help solve these problems. Companies can use real-time data and advanced analytics to improve their operations, reduce waste, and increase profitability. Here, we will explore how data-driven decision-making in manufacturing can make a huge difference in your operations and profitability.
Identifying the Common Pain Points in Manufacturing Operations
Before discussing the benefits of data-driven decision-making, let’s examine the key issues that many manufacturers face.
Operational Inefficiencies
Manufacturers often struggle with inefficiencies in their operations. For example, machinery might run too slowly, or workers may not be properly scheduled, leading to idle time and delays. Without accurate, real-time data, it’s hard to identify these problems in time to fix them, resulting in wasted resources and time.
High Operational Costs
As production costs rise, manufacturers are under constant pressure to reduce expenses. From rising labor costs to energy consumption, it can be difficult to identify areas where savings can be made without data-driven insights. In some cases, inefficient processes lead to excessive waste, further driving up costs.
Quality Control Challenges
Maintaining product quality is a major concern for manufacturers. Variability in product quality or defects can lead to returns, customer dissatisfaction, and increased rework costs. Data is crucial for identifying trends and pinpointing the exact point in the production cycle where quality begins to dip, allowing companies to correct issues before they result in defective products.
Supply Chain Disruptions
The global supply chain is fragile. Unexpected disruptions, such as material shortages or transport delays, can cause severe setbacks. Forecasting demand and managing inventory are some of the biggest challenges in manufacturing. Without data, predicting these issues is almost impossible.
Data Silos
In many companies, data is stored in different systems or departments. This creates data silos, where information from one department isn’t available to another. For example, the production team may lack visibility into inventory levels or customer demand. This lack of integration leads to poor decision-making and missed opportunities.
The Role of Data-Driven Decision-Making in Overcoming These Challenges
Now that we’ve identified the common pain points let’s explore how data-driven decision-making can address these challenges. By analyzing data from multiple sources, manufacturers can make smarter decisions and solve operational problems in real time real time.
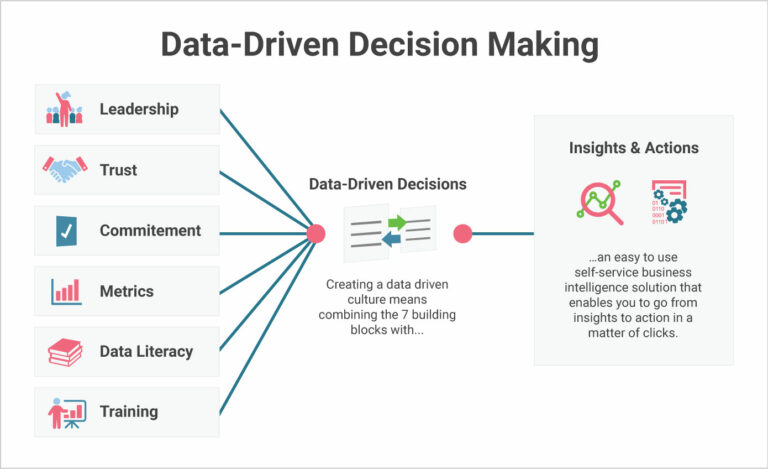
Real-Time Data Monitoring
One of the biggest advantages of data-driven decision-making is monitoring production processes in real time. Manufacturers can track key metrics such as cycle times, yield rates, and machine performance with sensors and IoT devices on the shop floor. This helps managers quickly identify challenges and take corrective action, reducing downtime and improving efficiency.
For instance, if a machine is about to fail, predictive analytics can alert the maintenance team before a breakdown occurs. By addressing problems before they become critical, manufacturers can avoid costly repairs and unplanned downtime.
Predictive Analytics for Cost Reduction
Data-driven decision-making also plays a key role in reducing operational costs. Predictive analytics helps manufacturers forecast demand, adjust production schedules, and optimize inventory levels. For example, if data indicates that a particular product will have high demand in the coming months, manufacturers can increase production in advance, reducing the risk of stockouts.
In addition, by analyzing historical data, manufacturers can optimize the use of raw materials, energy, and labor. This leads to fewer waste materials and lower energy consumption, contributing to significant cost savings.
Optimizing Resource Allocation
Effective resource allocation is a major challenge for manufacturers. Whether managing human resources, machinery, or raw materials, it’s essential to ensure that everything is being used efficiently. Data-driven decision-making can help optimize these resources.
For example, by analyzing data from production schedules, manufacturers can better allocate labor to the areas where it’s needed most, ensuring that production lines run smoothly. Data can also help schedule machine maintenance to prevent downtime during peak production times.
Improving Product Quality
Data-driven decision-making is also essential for maintaining and improving product quality. Through sensors and machine learning algorithms, manufacturers can continuously monitor the quality of products as they are being produced. Corrective actions can be taken immediately if any quality issues are found, preventing defects and cutting down waste.
Real-time data also allows for continuous quality improvement. Manufacturers can identify patterns and trends in the data, refining their processes over time. For example, if certain conditions consistently produce higher-quality products, these conditions can be repeated to improve overall output.
Enhancing Supply Chain Resilience
Managing a supply chain in today’s global market is more complex than ever. Disruptions from natural disasters, labor strikes, or material shortages can cause delays and increase costs. Data-driven decision-making helps mitigate these risks by providing greater visibility into the supply chain.
Manufacturers can use data to predict potential disruptions and proactively address them. For example, manufacturers can forecast material shortages by analyzing historical data and finding alternative suppliers in advance. This helps prevent costly delays and ensures that production continues without interruption.
Data Integration and Collaboration
Finally, data-driven decision-making eliminates data silos and improves collaboration across departments. By integrating data from different sources, manufacturers gain a comprehensive view of their operations, making it easier to make informed decisions.
For example, when production, procurement, and logistics teams share data, they can work together more effectively. This helps ensure that production schedules are aligned with material availability, reducing delays and improving overall efficiency.
Measuring the Impact of Data-Driven Decision-Making
One of the main reasons manufacturers adopt data-driven decision-making is its measurable impact on performance and profitability. By tracking key performance indicators (KPIs), manufacturers can assess the effectiveness of their data-driven strategies.
Key Performance Indicators (KPIs)
Some of the most important KPIs in manufacturing include:
- Throughput: The amount of product produced within a given timeframe. By optimizing processes using data, manufacturers can increase throughput.
- Defect Rate: The percentage of products that don’t meet quality standards. By using real-time data to monitor quality, manufacturers can reduce defect rates.
- On-Time Delivery: The percentage of orders delivered on time. Data can help improve scheduling and inventory management, resulting in more reliable delivery times.
- Cost Per Unit: The total cost of producing a unit of product. By reducing waste and improving efficiency, data-driven decision-making can help lower this cost.
Return on Investment (ROI)
The impact of data-driven decision-making can be seen in ROI. According to a report by McKinsey & Company, manufacturers who use data-driven technologies to optimize their operations see an average of 10-15% improvement in operational efficiency, along with significant cost savings.
One example is General Electric, which implemented predictive maintenance using data analytics. By predicting when equipment would fail, GE reduced maintenance costs by 25% and increased productivity by 10%.
Overcoming Data Management Challenges in Manufacturing
While data-driven decision-making offers significant benefits, managing data can be a challenge. Manufacturers must gather clean, relevant, and actionable data from various sources, including machines, sensors, and ERP systems.
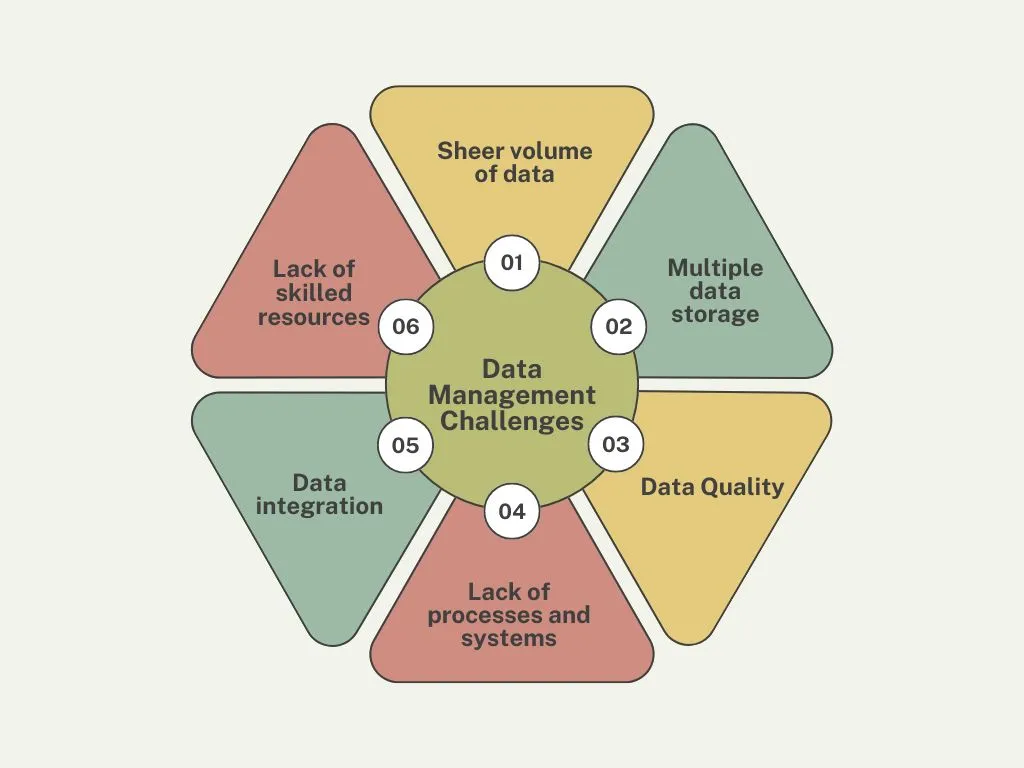
Data Collection and Integration
Manufacturers must collect data from multiple sources and integrate it into a single platform to make informed decisions. This can be difficult, especially if the data is stored in different systems or departments. However, by investing in data integration tools, manufacturers can centralize their data and gain a unified view of their operations.
Data Quality and Accuracy
The quality of the data is just as important as the quantity. Inaccurate data can lead to poor decision-making, so it’s important to ensure that data is clean, accurate, and timely. Regular audits & quality control checks can help maintain data integrity.
Data Security and Privacy
As manufacturers collect more data, they must also address data security and privacy concerns. Protecting sensitive information, such as production secrets and customer data, is essential. Implementing strong cybersecurity measures and adhering to privacy regulations will help safeguard data and maintain trust.
Frigate’s Data-Driven Solutions for Boosting Operational Performance
Frigate has positioned itself as a leader in applying data-driven decision-making to optimize manufacturing operations. Using cutting-edge technologies like IoT sensors, machine learning algorithms, and advanced data analytics, Frigate provides manufacturers with powerful tools to enhance performance, reduce operational costs, and drive profitability. Below, we will delve deeper into how each of these capabilities works and how they benefit manufacturers.
Real-Time Data Analytics
Real-time data analytics is one of the cornerstones of Frigate’s approach to data-driven manufacturing. With the use of Internet of Things (IoT) sensors and other connected devices placed throughout the production line, Frigate continuously collects data on key operational metrics, such as:
- Machine performance (e.g., speed, cycle time, uptime)
- Production throughput (units produced per hour)
- Energy consumption (for both machinery and facility operations)
- Temperature and humidity levels (critical for certain materials and processes)
- Vibration and sound data (which can be indicative of potential machine malfunctions)
This real-time data stream is sent to Frigate’s central analytics platform, where advanced algorithms process and interpret the data to detect trends, inefficiencies, and potential bottlenecks in production. For example, if a machine’s output drops below a certain threshold, the system can immediately alert the operators to investigate the cause. This proactive monitoring allows manufacturers to make adjustments in real-time, reducing downtime and optimizing production schedules.
Predictive Maintenance
One of the most impactful ways Frigate uses data-driven decision-making is through predictive maintenance. Rather than relying on scheduled maintenance intervals, which can either be too early (wasting resources) or too late (leading to unexpected breakdowns), predictive maintenance uses real-time machine data combined with historical performance data to predict when a piece of equipment will likely fail.
Frigate’s predictive maintenance system utilizes ML algorithms that are trained on huge amounts of historical equipment data, such as:
- Operating hours (e.g., how long each machine has been running since its last maintenance)
- Performance data (e.g., if a machine is performing below expected standards)
- Vibration patterns (which can indicate mechanical failure)
- Temperature anomalies (which could signal overheating)
By continuously monitoring these factors and analyzing them in real-time, Frigate can predict equipment failure well in advance, often before any visible signs of failure appear. This allows maintenance teams to address the issue during scheduled downtimes rather than risking unplanned shutdowns. This helps manufacturers cut maintenance costs, make machines last longer, and boost equipment reliability.
Optimized Resource Allocation
Frigate’s data-driven approach also helps manufacturers allocate resources more efficiently. By analyzing historical and real-time production data, Frigate’s platform can identify where resources (including labor, machines, and materials) are under or overutilized.
For instance, Frigate’s system can track:
- Labor efficiency by analyzing the time each operator spends on different tasks.
- Machine utilization rates, ensuring that machines are neither underused nor overburdened.
- Raw material usage allows manufacturers to pinpoint excess inventory or waste in production.
By comparing this data against optimal operational standards and historical performance benchmarks, Frigate can recommend adjustments to the scheduling of employees, equipment, and material orders. For example, if certain machinery is underperforming, the system might suggest increasing its maintenance frequency or reassigning it to less demanding tasks. Similarly, labor hours can be reallocated to high-demand areas, preventing overstaffing or understaffing in specific workstations.
Data-Driven Quality Control
In modern manufacturing, quality control isn’t just about conducting tests at the end of the production line. Frigate’s data-driven approach allows continuous product quality monitoring throughout production. By integrating advanced sensors and real-time analytics, Frigate helps manufacturers ensure that every product meets the required standards and issues are detected long before products leave the production floor.
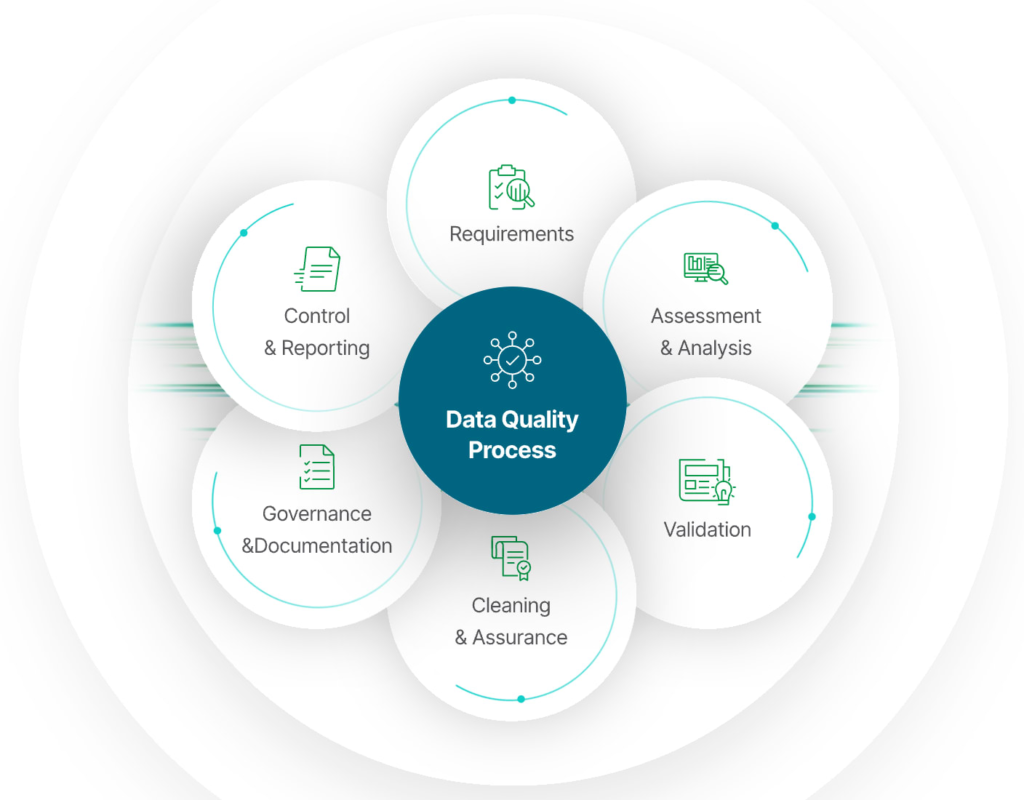
For example, Frigate utilizes vision systems and machine learning algorithms to continuously monitor the quality of each product. These systems can detect small defects that might otherwise go unnoticed, such as:
- Surface imperfections
- Incorrect dimensions
- Color variations
- Assembly errors
The quality data is analyzed in real-time and compared to predefined quality standards. If the system detects deviations from these standards, it can immediately alert the production team to take corrective action. This reduces the number of defective products, decreases the amount of rework required, and helps manufacturers avoid costly product recalls or returns.
Moreover, Frigate’s quality control systems aren’t limited to detecting defects—they can also identify root causes of quality issues. For example, if a specific machine consistently produces defective parts, the system might pinpoint issues such as misalignment, incorrect tool wear, or improper temperature settings. This insight allows manufacturers to make data-driven decisions to improve their processes and avoid future quality problems.
Integrated Supply Chain Management
Supply chain disruptions can have a devastating impact on manufacturing. Whether it’s a shortage of raw materials, transportation delays, or supplier issues, manufacturers need the ability to respond quickly to maintain production flow. Frigate’s data-driven decision-making capabilities extend to supply chain management, giving manufacturers greater visibility and control over their entire supply chain.
Frigate’s system integrates data from suppliers, logistics providers, and inventory management systems, allowing manufacturers to:
- Forecast demand more accurately using historical data, market trends, and predictive algorithms. This ensures that production lines are aligned with demand, reducing the chances of stockouts or overproduction.
- Monitor supplier performance, assessing reliability in terms of delivery times, quality, and cost. If a supplier is consistently late or delivers poor-quality materials, this information is automatically flagged, enabling manufacturers to seek alternative sources or address issues before they impact production.
- Track inventory levels in real-time, ensuring that raw materials are available when needed without excessive stockpiling. This reduces carrying costs and prevents stockouts.
Additionally, Frigate provides manufacturers with insights into potential supply chain disruptions. For example, suppose data from the logistics team shows that a shipment is delayed due to bad weather. In that case, the system can alert manufacturing teams so they can adjust production schedules or source materials from alternative suppliers.
Conclusion
Data-driven decision-making is revolutionizing the manufacturing industry. Manufacturers can improve efficiency, reduce costs, and enhance product quality by using real-time data and advanced analytics. The result is improved performance and higher profitability.
If your manufacturing processes could benefit from data-driven insights, Frigate’s expertise in this area can help. Contact Frigate today to learn how we can help you make smarter, data-driven decisions that will drive your business forward.